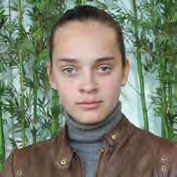
Alexandra Berg
MIT EECS | Quick Undergraduate Research and Innovation Scholar
Characterizing Robustness of Neural Networks for Histopathological Image Segmentation
2019–2020
Electrical Engineering and Computer Science
- AI and Machine Learning
- Graphics and Vision
Pawan Sinha
Digital histopathology, the machine-aided processing of human tissue samples, promises life-saving advances in the accuracy, efficiency, and scope of medical diagnostics. One of the field’s key goals is to automate “image segmentation”, the localization and classification of cellular entities, as this information is critical to many cancer grading schemes. Several neural networks have been proposed for this purpose – however, their robustness, or their capability of performing segmentation across different dataset conditions, is poorly understood. This project aims to synthesize images reflecting dataset degradation variations in order to characterize the robustness of top performing entries in the International Symposium on Biomedical Imaging’s 2016 international cancer metastasis detection and segmentation challenge (Camelyon16).
I am participating in SuperUROP because I want to engage in a long-term research project centered on machine vision-aided medical diagnostics and gain more exposure to multi-disciplinary collaborations within the healthcare R&D sector. I hope to use my previous UROP work in digital histopathology as a foundation for my project, which I dream might one day play some small, but genuine, role in helping another human being.