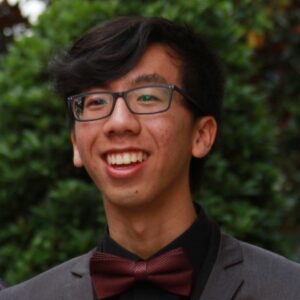
Alex Quach
Eric and Wendy Schmidt Center funded Research and Innovation Scholars
Generative Modeling Of Cellular Images For Fair And Explainable AI
2021–2022
EECS
- Computer Vision and Graphics
Caroline Uhler
Modern deep learning models are important to biological imaging; however, they are often not very interpretable and are prone to uncontrollable experimental noise (e.g., varying stain intensity or cell density). We aim to harness the power of generative models: 1) to provide meaningful interpretations of visual differences between biological images (explainability), and 2) to correct for nuisance factors of variation that could confound the correct interpretation of biological signals (fairness). Using style transfer models to transform cells to appear in one or another state, we hope to explain important visual patterns that differentiate populations of cells. Similarly, style transfer models can be used to correct for noise and experimental / instrumental variation present in images.
I am participating in SuperUROP because I want to work on a longer-term research problem. I have experience primarily in machine learning, so I am excited to extend my expertise into biological imaging. I hope to also dive deeper into generative modeling as well as interpretability and fairness. I look forward to formulating novel techniques that can be published as independent research.