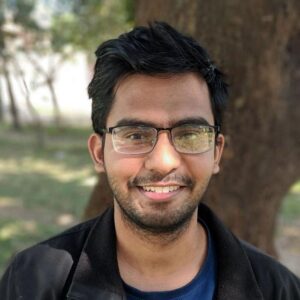
Adib Hasan
MIT Quest for Intelligence | Undergraduate Research and Innovation Scholar
Right for Right Reasons: Interpretably Robust NLP Models
2020–2021
EECS
- Natural Language and Speech Processing
Regina A. Barzilay
Adversarial attacks on deep neural networks is a growing area of concern in machine learning. In natural language processing, the attacks come in the form of intentionally perturbed text input that seem innocuous to the humans but misdirect the models. This has cascading effects from reducing the accuracy to jeopardizing the reliability of the predictions. In our research, we shall investigate an interpretability driven defense mechanism against adversarial attacks. We shall search for ways to train models so that their decisions are based on human interpretable causal factors. This will enable the models to make more human-like predictions. Consequently, they will also be resilient to adversarial attacks that will not fool humans.
I am very interested in deep learning research. This SuperUROP presents me an opportunity to gain more experience in this field, as well as make a positive contribution to my group. Previously, I have taken machine learning courses such as 6.867 and 6.864 and I want to expand on that knowledge with real‐world applications. I hope to publish a paper by the end of the SuperUROP, if I have meaningful results to display.