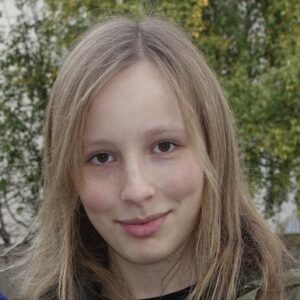
Adeline Hillier
MIT Quest for Intelligence | Undergraduate Research and Innovation Scholar
Leveraging Machine Learning to Transform Climate Modeling
2020–2021
EAPS
- Environment
Raffaele Ferrari
Climate models rely on subgrid-scale models to represent turbulent dynamics that occur at scales finer than the model grid. Although the small-scale dynamics can be derived from fluid dynamical equations, parameterizations approximating their effects are necessary because explicitly resolving the dynamics is computationally intractable at high resolutions. I will apply ML techniques to develop parameterizations of penetrative convection in the upper ocean. The convection case study will provide insight into whether the ML approaches studied Gaussian process regression, equation discovery, and neural networks are well-suited to the task of representing real-world ocean dynamics with uncertainty quantification; if so, they may prove themselves worthy of incorporation into global models.
I look forward to pursuing environmentally-motivated research that will allow me to dip my toes in computational fluid dynamics, write code in Julia, and engage with some of the practical trade-offs involved in designing parameterization schemes. Above all, I will be thrilled to get to interact with, and gain intuitions from, CliMA scientists and graduate students who have mastered their domains. What more could I wish for?