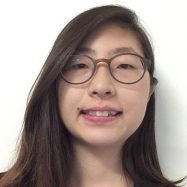
Abigail Choe
MIT EECS | Undergraduate Research and Innovation Scholar
Use of Diversity for Effective Ensemble Modeling
2016–2017
Electrical Engineering and Computer Science
- AI and Machine Learning
Una-May O'Reilly
With the rise of cloud services, resources from all over the world can now be easily compiled. In the field of machine learning and evolutionary computation, that has prompted the development of FCUBE, a cloud machine-learning framework for automatic deployment of existing learning algorithms. FCUBE runs all the learners on a given data set and returns a merged model. Howeer, such an approach may be ineffective and time-consuming due to bias from weak or overlapping learners. This problem will become more serious as the number of learners on FCUBE increases and hinder its performance significantly. Therefore, this project will develop a better merge algorithm that will fuse the models selectively based on individual learners’ performance and the diversity of the set of learners to be combined.
I have done two internships in the past, both of which I really enjoyed. They provided me with a dynamic but friendly environment where I was challenged and grew as a result. During the semester, I missed being in such an environment and decided to participate in SuperUROP so that I can continue to learn as well as contribute to a bigger project outside of the classroom.