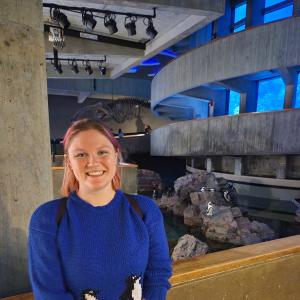
Zoe Anne Gotthold
Undergraduate Research and Innovation Scholar
Machine Learning can Predict Translation Efficiency in Toxoplasma gondii
2023-2024
Electrical Engineering and Computer Science
- Artificial Intelligence for Healthcare and Life Sciences
Sebastian Lourido
Toxoplasma gondii is a ubiquitous parasite among warm-blooded animals that can cause both acute and chronic infections (toxoplasmosis). Symptoms can be particularly severe in immunocompromised individuals. After an acute infection, Toxoplasma can differentiate into long-lasting stages known as bradyzoites. Previous research has noted the importance of translational regulation in this Toxoplasma life cycle: in particular, BFD1, the master regulator of Toxoplasma differentiation, is translationally, rather than transcriptionally, controlled.
Our research focuses on understanding translational control in Toxoplasma through the creation of machine-learning models. Using ribosome profiling, we analyzed the specific RNAs bound by ribosomes, known as ribosome footprints. Normalizing the number of ribosome footprints to the total number of mRNAs provides a quantitative measure of translation efficiency for each gene.
Using a random-forest model trained on several parameters, including coding sequence length, upstream start codon data, GC content, and UTR lengths, we were able to generate a model of translation efficiency (on unseen data, R2 = 0.42, Pearson’s correlation = 0.65). Interestingly, this model is much less predictive in human data sets (R2 = 0.21) since a model trained on human fibroblasts places higher importance on GC content and 5’ UTR length than the Toxoplasma model. This could indicate the unique role of the 5′ UTRs in Toxoplasma, where specific UTR features might matter more than 5′ UTR length.
We also trained several more unsupervised machine learning models on only the sequences of Toxoplasma transcripts. Classifying each gene as ‘high’ or ‘low’ in terms of translation efficiency, we were able to train an effective LSTM (long short-term memory) network on sequence and length data alone (AUC=0.76). These models will allow us to better understand the translational level of genetic regulation, a regulation which seems to be critical for parasite persistence in the host.
I took SuperUROP because I am looking to develop my computational skills. As someone who plans to study infectious diseases, I believe that it is important to learn how we can use computational power to enhance research. We are in an evolutionary arms race with pathogens, and one tool we have that they do not is the ability to code: we should use it!