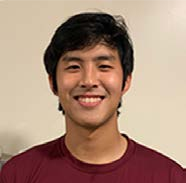
Kevin Shao
MIT EECS | Aptiv Undergraduate Research and Innovation Scholar
Efficient Deep Learning for Autonomous Driving
2021–2022
EECS
- Artificial Intelligence and Robotics
Song Han
In a typical autonomous driving stack, the first module is the perception system. Several modalities are possible, but the richest information can be obtained through LiDAR scans, which give a 3D view of the scene. It is critical in common tasks, such as object detection, to be effective and efficient. Our research will focus on efficiency both annotation efficiency and computational efficiency. In particular, we will focus on the temporal domain an especially information-rich area. While most research focuses on single-frame processing, we will explore algorithms for processing sequential frames in order to either boost computational efficiency or perform unsupervised learning for annotation efficiency. We hope this project will help move toward real-time fully autonomous driving.
Through SuperUROP, I hope to gain more experience in autonomous vehicle research, as well as help out in the HAN Lab, which has already taught me a lot. I’m also excited to apply the theoretical knowledge from machine learning courses to this real-life, tangible domain. Being a member of the MIT Driverless team as well, I’m hoping to delve even deeper into the world of self-driving cars.