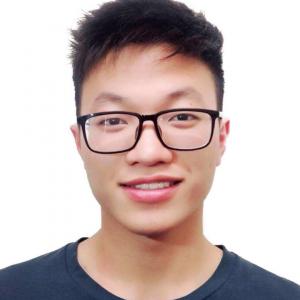
Xin Yu Lin
MIT EECS | Mason Undergraduate Research and Innovation Scholar
Understanding the Limits of Machine and Human Vision
2020–2021
EECS
- Artificial Intelligence and Machine Learning
Boris Katz
ObjectNet is a new adversarial object recognition test set that has been used to demonstrate a 40-45% decrease in performance on current models from VGG to ResNet. ObjectNet is challenging because it contains controls for backgrounds, rotations, and viewpoints. The goal of our project is to understand the limits of the existing object recognition models and why they perform poorly on ObjectNet. In particular, the project will involve building better object recognition models, gathering human object recognition data, evaluating the results of human and machine experiments against previous ObjectNet benchmarks, and identifying/quantifying interesting patterns in ObjectNet images that affect object recognition performance for both new machine models and for humans.
I’m participating in SuperUROP because I want to gain a more well-rounded experience in computer vision research. I’ve taken courses in machine learning, algorithms, and signal processing, and I look forward to applying some of those skills to real-world research problems. Through SuperUROP, I hope to have meaningful results to display.